In a paper published Thursday in the journal Science, researchers at New York University report that AI, given just a tiny fraction of the fragmented experiences of one child, can begin to discern order in the pixels, learning that there is something called a crib, stairs or a puzzle and matching those words correctly with their images.
The tool the researchers used is not an AI that learns just like a child. But the research shows that AI can pick up some basic elements of language from the sensory input of a single child’s experience, even without preexisting knowledge of grammar or other social abilities. It’s one piece of a much larger quest to eventually build an AI that mimics a baby’s mind, a holy grail of cognitive science that could help researchers understand our own development and lead to AI that humans could teach new skills in a more intuitive way.
Chatbots, also known as “large language models,” demonstrated that AI trained on massive amounts of text can produce a voluble conversation partner with a dazzling mastery of language. But many cognitive scientists contend that this verbal feat falls short of actual human thinking.
Babies are the opposite of a chatbot, learning words not by rapidly digesting all the world’s texts, but from being in the world itself, through sensory input and play.
“By our calculations, it would take a child 100,000 years of listening to spoken words to reach the word count” of the training sets for chatbots, said Brenden Lake, a computational cognitive scientist at NYU who led the study. “I was also skeptical that those [chatbot] models would shine a lot of light on human learning and development.”
Linguists, philosophers, cognitive scientists and — increasingly — AI developers have all been puzzling over how humans learn language.
For years, scientists have been trying to understand how children’s minds take shape through carefully controlled experiments. Many involve toys or puppets that allow researchers to probe when various cognitive skills come online. They’ve shown that 16-month-old babies can deploy statistical reasoning to determine whether a noisemaker is broken, and that babies as young as 5 months know that an object still exists even when they can’t see it, a key developmental milestone called object permanence.
In addition, some individual babies have been closely followed over time. Deb Roy, a scientist at the Massachusetts Institute of Technology, set up overhead cameras in all the rooms of his house in 2005 and recorded his son’s linguistic development, providing a massive trove of data that chronicled the acquisition and evolution of words. That work suggested it was not how many times a word was repeated that predicted whether Roy’s son learned it early, but whether it was uttered in an unusual spot in the house, a surprising time or in a distinctive linguistic context.
The innovative use of headcams has given researchers an even more intimate view of early childhood.
Since 2013, several families have contributed to the SAYCam database, a collection of audiovisual recordings from individual babies and toddlers over a crucial period of cognitive development, between 6 and 32 months. Families of the babies, who are identified only by first name, put cameras mounted on headbands on their children for about two hours a week.
Scientists can apply for access to the data, which provides a unique window into each child’s world over time and is intended to be a resource for researchers across a variety of fields.
Sam, whose identity is private, is now 11 years old. But the recordings of his early life in Australia provided Lake and his colleagues with 600,000 video frames paired with 37,500 transcribed words of training data for their AI project.
They trained their relatively simple neural network on data captured when Sam was between the ages of 6 months and 2 years. The AI, they found, learned to match basic nouns and images with similar accuracy to AI trained on 400 million images with captions from the web.
The results wade into, but don’t solve, a long-running debate in science about the basic cognitive skills humans need built into their brains to learn language.
There are various theories of how humans learn language. High-profile linguist Noam Chomsky proposed the idea of a built-in, innate language ability. Other experts think we need social or inductive reasoning skills for language to emerge.
The new study suggests that some language learning can occur in the absence of specialized cognitive machinery. Relatively simple associative learning — see ball, hear “ball” — can teach an AI to make matches when it comes to simple nouns and images.
“There’s not anything inbuilt into the network giving the model clues about language or how language ought to be structured,” said study co-author Wai Keen Vong, a research scientist at NYU.
The researchers don’t have comparable data on how a 2-year-old would perform on the tasks the AI faced, but they said that the AI’s abilities fall short of those of a small child. For instance, they could track where the AI was focusing when prompted with various words and found that, while it was spot-on for some words such as “car” or “ball,” it was looking in the wrong area when prompted with “cat.”
“I want to find out the minimal ingredients needed to build a model that can learn more like a child does — this is a step,” Lake said.
The rudiments of language
The AI picked up its vocabulary of objects from being exposed to 1 percent of Sam’s waking hours — 61 hours of footage accumulated over a year and a half. What intrigued outside scientists about the study was both how far the AI got based on that, and how far it still had to go to recapitulate human learning.
“It’s really important and new to be applying these methods to this kind of data source, which is the data from a single child’s experience, both visual and auditory,” said Joshua Tenenbaum, a computational cognitive science at MIT who was not involved in the work.
“What I would add is there are still some things it’s harder to conclude from the paper exactly — what this tells us about how children actually learn words is less clear.”
Michael Tomasello, a developmental and comparative psychologist at Duke University, said that the AI model might reflect how a dog or a parrot can learn words. Experiments show that some dogs can learn more than 100 words for common objects or stuffed animals.
But, he pointed out, it remains unclear how this AI could take sensory input and glean verbs, prepositions or social expressions.
“It could learn that a recurrent visual pattern is ‘doll’. But how does it learn that that very same object is also a ‘toy’? How does it learn ‘this’ or ‘that’ or ‘it’ or ‘thing’?” Tomasello wrote in an email.
The AI model trained on the child’s experience, he noted, was able to identify things that can be seen, and that’s just a small part of the language that children hear and learn. He proposed an alternative model, where instead of simply associating images with sounds, an AI would need to make inferences about the intention of communication to learn language.
Lake is starting to train AI models on video instead of still frames to see if they can successfully expand their vocabulary into verbs and abstract words. There will soon be an additional stream of data to work from, because Lake is collecting data from his young daughter.
But he acknowledged the ways the AI learns deviate from children’s learning, even for simple words. The AI was really great at learning to identify sand, for example, but had trouble with hands, which means its progress probably does not reflect most children’s grasp of their environment.
“’Sand’ was too easy, ‘hand’ was too hard,” Lake said. “And, the model doesn’t know that milk and pears tastes good.”
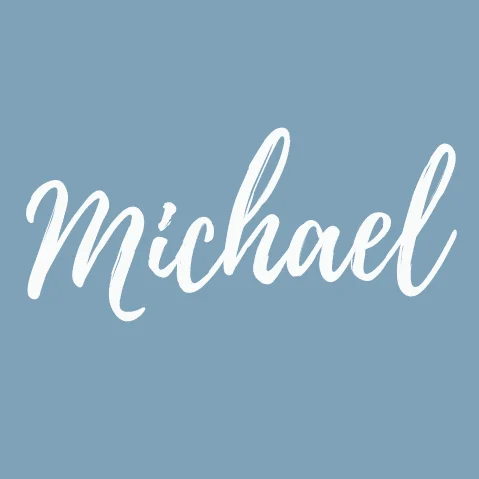
Michael Johnson is a tech enthusiast with a passion for all things digital. His articles cover the latest technological innovations, from artificial intelligence to consumer gadgets, providing readers with a glimpse into the future of technology.