Summary: Researchers mapped disease trajectories from birth to death, analyzing over 44 million hospital stays in Austria to uncover patterns of multimorbidity across different age groups.
Their groundbreaking study identified 1,260 distinct disease trajectories, revealing critical moments where early and personalized prevention could alter a patient’s health outcome significantly. For instance, young men with sleep disorders showed two different paths, indicating varying risks for developing metabolic or movement disorders later in life.
These insights provide a powerful tool for healthcare professionals to implement targeted interventions, potentially easing the growing healthcare burden due to an aging population and improving individuals’ quality of life.
Key Facts:
- Mapping Multimorbidity: The study identified 1,260 disease trajectories, underscoring the prevalence of multimorbidity and highlighting opportunities for early intervention.
- Critical Moments Identified: Analysis revealed crucial points where disease paths diverge, suggesting targeted prevention could significantly impact future health outcomes.
- Personalized Prevention: The research underscores the importance of early, personalized healthcare strategies to mitigate long-term health risks and reduce the burden on healthcare systems.
Source: CSH
The world population is aging at an increasing pace. According to the World Health Organization (WHO), in 2023, one in six people were over 60 years old. By 2050, the number of people over 60 is expected to double to 2.1 billion.
“As age increases, the risk of multiple, often chronic diseases occurring simultaneously—known as multimorbidity—significantly rises,” explains Elma Dervic from the Complexity Science Hub (CSH). Given the demographic shift we are facing, this poses several challenges.
On one hand, multimorbidity diminishes the quality of life for those affected. On the other hand, this demographic shift creates a massive additional burden for healthcare and social systems.
Identifying typical disease trajectories
“We wanted to find out which typical disease trajectories occur in multimorbid patients from birth to death and which critical moments in their lives significantly shape the further course. This provides clues for very early and personalized prevention strategies,” explains Dervic.
Together with researchers from the Medical University of Vienna, Dervic analyzed all hospital stays in Austria between 2003 and 2014, totaling around 44 million. To make sense of this vast amount of data, the team constructed multilayered networks. A layer represents each ten-year age group, and each diagnosis is represented by nodes within these layers.
Using this method, the researchers were able to identify correlations between different diseases among different age groups — for example, how frequently obesity, hypertension, and diabetes occur together in 20-29-year-olds and which diseases have a higher risk of occurring after them in the 30s, 40s or 50s.
The team identified 1,260 different disease trajectories (618 in women and 642 in men) over a 70-year period. “On average, one of these disease trajectories includes nine different diagnoses, highlighting how common multimorbidity actually is,” emphasizes Dervic.
Critical moments
In particular, 70 trajectories have been identified where patients exhibited similar diagnoses in their younger years, but later evolved into significantly different clinical profiles.
“If these trajectories, despite similar starting conditions, significantly differ later in life in terms of severity and the corresponding required hospitalizations, this is a critical moment that plays an important role in prevention,” says Dervic.
Men with sleep disorders
The model, for instance, shows two typical trajectory paths for men between 20 and 29 years old who suffer from sleep disorders. In trajectory A, metabolic diseases such as diabetes mellitus, obesity, and lipid disorders appear years later. In trajectory B, movement disorders occur, among other conditions.
This suggests that organic sleep disorders could be an early marker for the risk of developing neurodegenerative diseases such as Parkinson’s disease.
“If someone suffers from sleep disorders at a young age, that can be a critical event prompting doctors’ attention,” explains Dervic.
The results of the study show that patients who follow trajectory B spend nine days less in hospital in their 20s but 29 days longer in hospital in their 30s and also suffer from more additional diagnoses. As sleep disorders become more prevalent, the distinction in the course of their illnesses not only matters for those affected but also for the healthcare system.
Women with high blood pressure
Similarly, when adolescent girls between the ages of ten and nineteen have high blood pressure, their trajectory varies as well. While some develop additional metabolic diseases, others experience chronic kidney disease in their twenties, leading to increased mortality at a young age.
This is of particular clinical importance as childhood hypertension is on the rise worldwide and is closely linked to the increasing prevalence of childhood obesity.
There are specific trajectories that deserve special attention and should be monitored closely, according to the authors of the study.
“With these insights derived from real-life data, doctors can monitor various diseases more intensively and implement targeted, personalized preventive measures decades before serious problems arise,” explains Dervic.
By doing so, they are not only reducing the burden on healthcare systems, but also improving patients’ quality of life.
About this health and AI research news
Author: Eliza Muto
Source: CSH
Contact: Eliza Muto – CSH
Image: The image is credited to Neuroscience News
Original Research: Open access.
“Unraveling cradle-to-grave disease trajectories from multilayer comorbidity networks” by Elma Dervic et al. npj Digital Medicine
Abstract
Unraveling cradle-to-grave disease trajectories from multilayer comorbidity networks
We aim to comprehensively identify typical life-spanning trajectories and critical events that impact patients’ hospital utilization and mortality. We use a unique dataset containing 44 million records of almost all inpatient stays from 2003 to 2014 in Austria to investigate disease trajectories.
We develop a new, multilayer disease network approach to quantitatively analyze how cooccurrences of two or more diagnoses form and evolve over the life course of patients. Nodes represent diagnoses in age groups of ten years; each age group makes up a layer of the comorbidity multilayer network.
Inter-layer links encode a significant correlation between diagnoses (p < 0.001, relative risk > 1.5), while intra-layers links encode correlations between diagnoses across different age groups. We use an unsupervised clustering algorithm for detecting typical disease trajectories as overlapping clusters in the multilayer comorbidity network.
We identify critical events in a patient’s career as points where initially overlapping trajectories start to diverge towards different states. We identified 1260 distinct disease trajectories (618 for females, 642 for males) that on average contain 9 (IQR 2–6) different diagnoses that cover over up to 70 years (mean 23 years).
We found 70 pairs of diverging trajectories that share some diagnoses at younger ages but develop into markedly different groups of diagnoses at older ages. The disease trajectory framework can help us to identify critical events as specific combinations of risk factors that put patients at high risk for different diagnoses decades later.
Our findings enable a data-driven integration of personalized life-course perspectives into clinical decision-making.
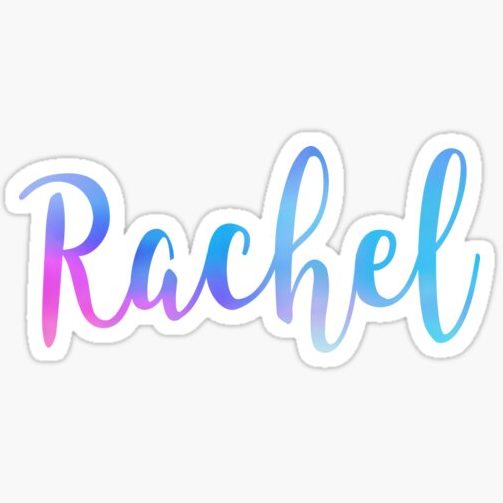
Rachel Carter is a health and wellness expert dedicated to helping readers lead healthier lives. With a background in nutrition, she offers evidence-based advice on fitness, nutrition, and mental well-being.